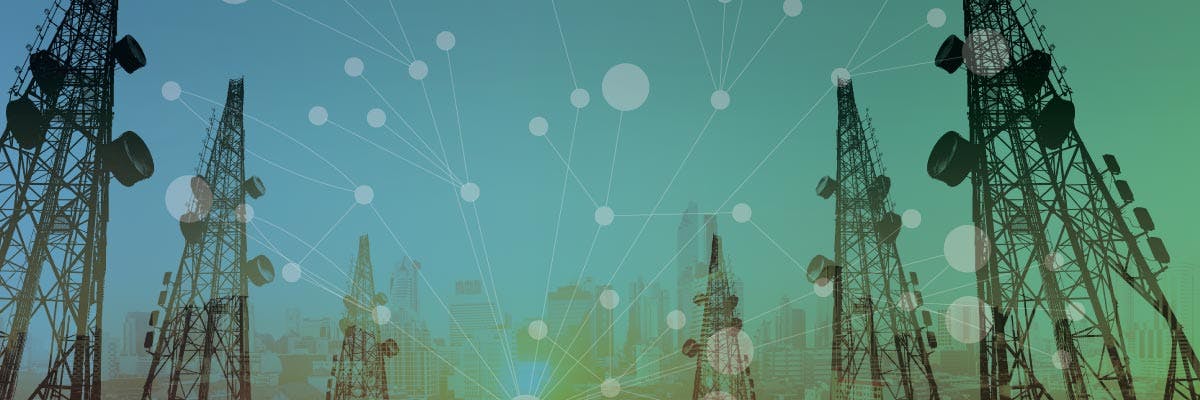
Predicting Network Performance
How Daisho's ML platform helped transform field services and network maintenance for a Telco by predicting network faults 5 hours in advance
What Daisho did
Daisho built network performance prediction models which were 93% accurate in predicting key performance metrics five hours in advance, and automatically identified cell towers which needed to be serviced by field engineers even before an issue got raised. Daisho’s Signal Factory identified key time-series patterns of performance indicators like traffic, seizures, drops, etc. for each cell tower, hours before an issue happened, making resolutions faster and easier.
What the Customer Wanted
Using Machine Learning to Predict Telecom Network Performance
Telecom networks are managed under strict service level agreements of performance of key network metrics e.g. call-setup success rate (CSSR) and call drop rate (CDR) must be above 99.99% and below 0.01% respectively. Every time such a metric falls below a specified ideal threshold, there is a potential hardware issue for which field engineers need to be dispatched. Getting the data to identify the root cause of the issue, and fixing an issue on the field takes about 5 hours. Hence, the problem was to predict 5 hours in advance, the likelihood that a cell tower’s CSSR and CDR was going to miss the SLA. Cell towers can then be ranked by their likelihood of breach and prioritised for resolution by the operations team 5 hours in advance.
How Daisho Solved the Problem
Daisho was provided two separate datasets - an hourly time-series performance data over four months of all cell towers operating in a network, and network topology data of the cell towers. The performance indicators covered traffic, seizures, drops, requests etc. which track all M2M communication between cell towers and mobile devices in the network. The topographic data on cell towers included network specifications as well as location information in terms of latitude and longitude of the towers.
Daisho’s SignalFactory built more than 3,500 signals from this data. Based on these, it was able to filter out two groups of signals which were highly predictive of performance issues of a cell tower in the future.
Historical performance of a cell tower
The Signal Factory scanned thousands of signals by looking at every possible time-window from as small as a minute to as far back as months back in the historical data for all provided indicators, before shortlisting a few for predictive signals. There were three types of performance signals which were identified automatically by Daisho - average metrics in the last 4 hours, 8 hours and 24 hours, a comparison of the cell’s current indicators to its recent or long-term historical performance till that time, and hours since its last ideal or non-ideal performance.
These signals were able to identify cells which have been performing below standards for a long period of time (and probably overlooked for very justifiable business reasons e.g. rural areas with very low traffic), as well as cells which were healthy but showing signs of strain (and which was more important for the business to look at e.g. fast growing business districts).
Neighbourhood based signals
Neighbourhood based signals identify how a cell is performing compared to its immediate spatial neighbours. This is particularly important in telecom since network load is redistributed to a cell tower's neighbours when traffic increases. A neighbourhood on the Daisho platform is a geographical boundary with varying levels of granularity -- level 1 is the whole earth, and level 30 typically corresponds to an area of size ~ 0.93 sq. cm. The Signal Factory scanned through all possible levels of granularity of a cell’s neighbourhood before shortlisting multiple neighbourhood traffic signals which were predictive of network performance.
Operationalising models on the Insight Jedi Platform
Daisho’s models and prediction workflows were deployed for operators in Europe and Asia. Daisho constantly auto-learns from an hourly data feed to monitor cells and shortlist the ones most likely to have issues. Daisho also provided key pointers to resolve the issues. Using interpretable models, the client was also able to figure out cells which could be fixed by the field engineers immediately and which need to be prioritised for later.