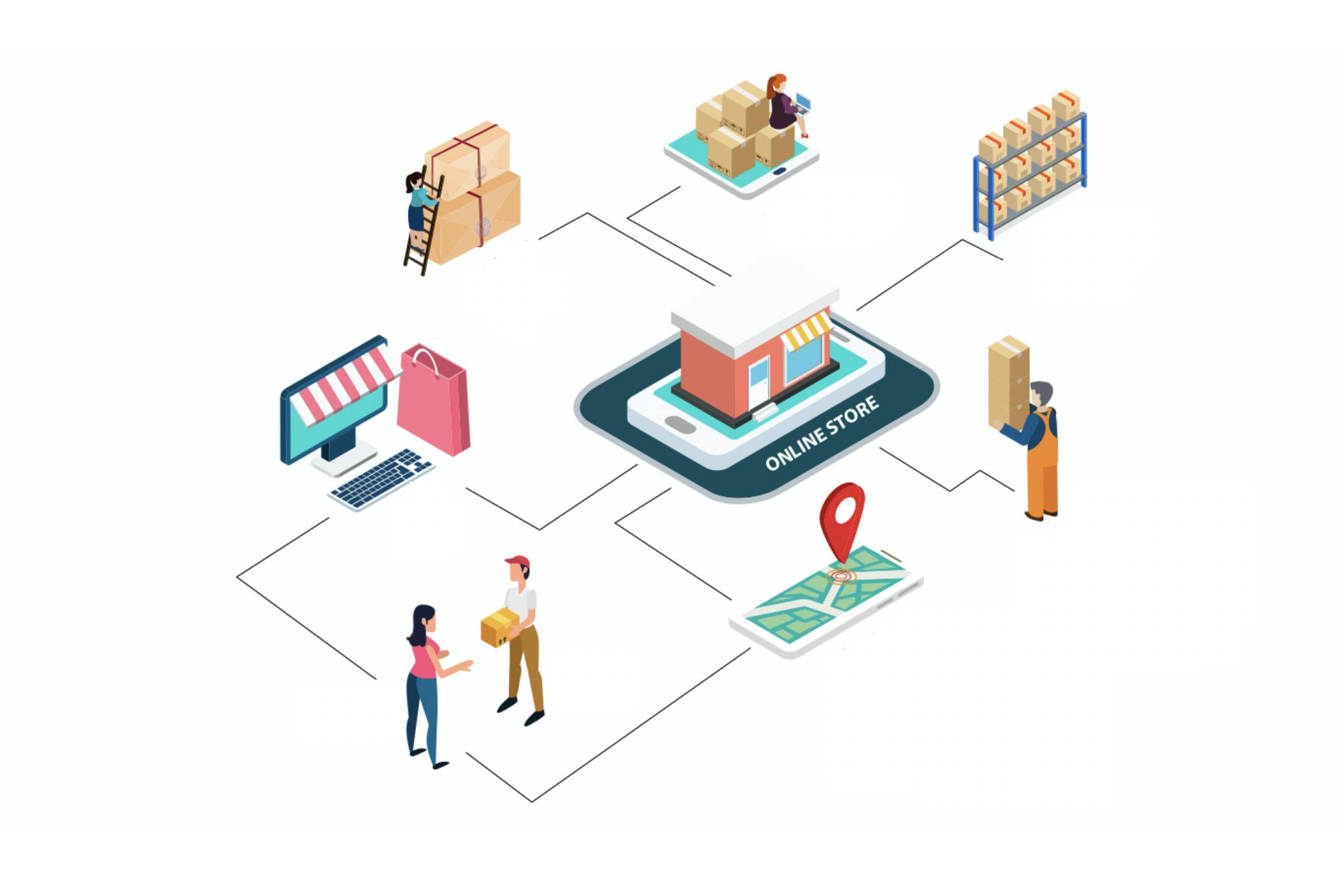
Impact of Late Deliveries on Customer Churn
How Daisho’s Signal Factory connected supply chain metrics with customer churn to prioritise order deliveries
What Daisho did
A large online apparel retailer wanted to understand how late deliveries were impacting customer churn, with customer churn being defined as a customer not making a transaction in the next 90 days. Daisho built uplift models connecting data from customer transactions and supply chain to prioritise deliveries based on increased risk of a customer churning due to a late delivery. This is the first time that data from these two functional groups in the company was utilised to provide a data-driven, consistent strategy.
The Problem
Data stuck in silos
How late delivery impacts customer retention metrics e.g. repeat buying behaviour, was not well understood by the business. A primary reason that such problems get overlooked is because data sits in two silos - customer management and supply chain. Each business team is well versed with the hows and whys of their own data, but insights connecting them is unknown territory for both sides.
For example, at a basic level, it was expected that customers churn more for late deliveries of some items than others, and seasons play an important role -- but the exact characteristics of orders and customers, and their interplay was not known.
How Daisho Solved the Problem
The Signal Factory approach to connecting silos
On one side, there was data on customer orders over almost two years covering where the orders came from, types of orders (whether it was a gift, if it was under a promotion, discounts, etc.), and metrics related to the shipping method chosen by the customer. On the other hand, there was the supply chain data covering metrics related to carriers, distribution centres and containers (size, weight of the shipped containers, etc).
On the overall data, the usual suspects failed to surface any actionable insights. Customers churned about 2 percent more if they received a late delivery and this behaviour did not vary at different levels of discounts. It was similar for order sizes -- incremental churn for late deliveries was constant for different order sizes. All hypotheses based on gut feelings failed.
Enter the Signal Factory. It built deep signals from the two disparate data sources, and using Daisho’s sophisticated machine-learning algorithms, the client was able to identify the complex interplay between the signals. Three big insights emerged:
1. Seasonality (peak vs off-peak seasons) was an important factor -- how customers reacted in the peak season is very different from the off-peak season. But it wasn’t the same for all customers or orders! For loyal customers or customers with non-standard orders, seasonality had no effect.
2. Although discounts had no impact on incremental churn during the peak season, customers were less forgiving in the off-peak season. Late deliveries of lower discounted items would draw more ire from customers than higher discounted items.
3. This punishing behaviour of the customer varied with the number of shipments -- late delivery of a single shipment would be much worse (in terms of incremental churn rates) than if the order had multiple shipments.
Operationalising the Models
The Signal Factory was able to connect customers and orders data to shipping data from the supply chain and build a model. The model output was effectively translated to an operations blue book to prioritise deliveries based on the incremental risk of a customer churning compared to an on-time delivery.