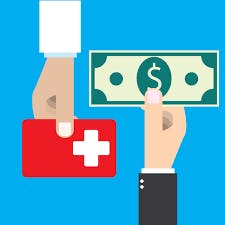
Call Prioritisation in Healthcare Collections
How Daisho’s Machine-Learning Automation Platform Supercharged a Small Analytics Team
What Daisho Did
Using Daisho’s ML automation platform, a 4-member analytics team built more than 40 models and delivered more than $3M in incremental annual commissions -- an improvement of 30% over the previous year! Automating the complete data science workflow -- cleaning data, testing thousands of hypotheses, building models and generating insights for business stakeholders, setting up weekly predictions -- was accomplished in minutes on the platform. More than 40 models were built in less than three months by a small team of analysts -- a 20X efficiency for the team!
What the team was aiming for
Collecting a patient's out-of-pocket spend is a very important part of revenue management for hospitals. The earlier a service representative targets a patient who has a higher propensity to pay his balance, the better the hospital will be in managing its revenue. The customer wanted to completely revamp their collections strategy in healthcare by replacing existing heuristics with machine-learning (ML) driven scores for prioritising calls.
However, every hospital chain was different - different regions, different patient profiles, different specialisations - and had to be modelled separately. The team took 4 months to build 1 model (for one hospital chain) using R. And they had a portfolio of about 100 hospital chains. This was clearly too slow, and they were looking to supercharge the process.
How the Team leveraged Daisho
About 40 hospitals out of more than 100 hospitals, responsible for most of the revenues, were chosen by the team. Each hospital had different kinds of data; but using Daisho’s EDA and auto-cleanup features, analysts quickly understood the data and cleaned it for model building.
Existing heuristics used for collections were also missing nuances. Hospitals which are run almost like nonprofits had very different patients responding to collections calls, compared to hospitals with more aggressive revenue management. Similarly, patients who have been sent to collections only recently, responded very differently to calls compared to patients who have been in collections for a longer time. Daisho was able to automatically extract the right signals for each hospital chain, solely from the data.
Using Daisho, analysts were also able to bust a lot of business biases. For example, Transunion score of a patient was expected to be an important indicator for her propensity to pay out-of-pocket spends. Similarly, the business expected special ZIP codes to be correlated with payment rates. But analysts generated insights from the platform which clearly proved that it wasn’t the case for all hospitals.
Operationalizing the Models
The team now maintains a set of models. As new collection requests come in, the team uses Daisho's action-engine to score each request in terms of likelihood to pay up. This is then sent to the callers, so that they can prioritise their calls accordingly.
In addition, the team re-trains models on a monthly basis using Daisho's auto-learn features. This ensures that all models are kept up-to-date, and all information is part of the model.
All this is being managed by a team of just 4 analysts.