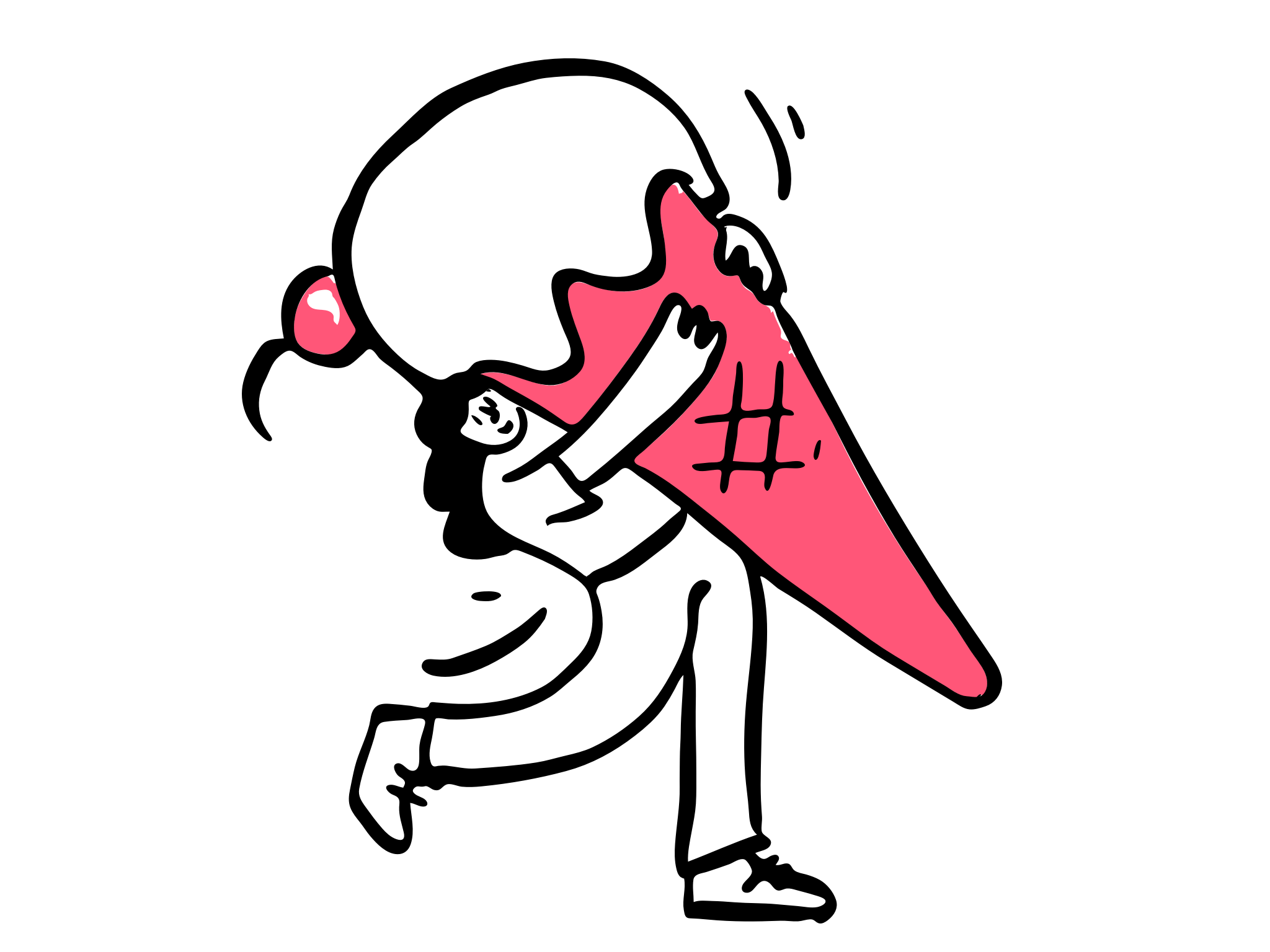
What's Gotten Worse (or Better) due to Covid-19
How A Small Team of Analysts Quickly Responded to the Covid-19 Pandemic using Daisho’s Automated Uplift Recipes by building more than 40 models inside 3 days.
The Context
Our customer is one of the largest BPO service providers, serving FTSE 100 & Fortune 500 companies across almost 40 delivery centers globally. Its healthcare division provides collections related services to hospitals. Its service representatives call the patient to either ask for payments for their out-of- pocket spend, or provide payment plans which might be favorable to the patient. A small team of four analysts support all machine-learning needs of the healthcare division using Daisho.
Covid-19 Pandemic and Impact on Healthcare Collections
The customer has seen a drop of 10 to 20 percent in the number of accounts coming up for collections in March and April 2020 compared to the same period last year. This is partly due to fewer hospital visits or deferrals - according to one survey of leaders in healthcare [1], 48 percent of responders expect a decline of 5 percent or more in insurance utilization. A lot of hospitals are also holding their collections inventory for favorable economic, social and legislative climate in the next couple of months resulting in fewer accounts for collections [2].
Not only that, based on March and April 2020 data, there has been a drop in average healthcare collections (per patient) between 5 percent and 70 percent compared to pre-covid rates amongst hospital networks it serves, with most of the decline in the 15 - 40 percent range. One reason is the uncertainty on the economic front which is making people more cautious about how much they finally end up paying for their out-of-pocket expenses -- in the same survey 78 percent of respondents expect a peak unemployment rate of 20% in the coming months [1].
But this masks key takeaways.
Daisho's models were able to uncover clusters, where there has been an increase of about 30-65 percent in average collections (per patient) post-covid. Patients using Medicare and Medicaid for payments have seen an increase where hospital visits were for recurring, inpatient and observation services related to pain management, urology, cardiac surgery and gastroenterology. Higher collection payments seem to be driven by higher out-of-pocket expenses, which are in turn due to inflated costs of medical supplies, and expenses towards keeping patients and staff safe in post-covid times [2].
While average collections have mostly dropped, there are a few key insights for specific hospitals which surfaced. Collections from patient visits for nasal endoscopies, minor surgeries involving removal of subcutaneous tissue, muscular tests, etc. have gone down about 20 percent from pre- covid times. Collections from unemployed and disabled are lower, and collections from emergency visits have also reduced.
Fast, Early, and Deep Insights From Automated Uplift Recipes
The current healthcare collections strategy for our client is driven by data; predictive scores from more than 40 machine-learning models built on Daisho are used to prioritize calls based on their propensity to pay. Each hospital network comes with its own community focus and outlook not only towards healthcare but also on how patients with pending payments are treated, and hence need to be modelled separately.
Using Daisho, a small team of 2 analysts were able to build individual models for all 40 hospital networks in just 3 days.
They used the generic uplift-model recipe on Daisho to accomplish this. Uplift models are a class of predictive models which identifies pockets of uplift (positive or negative) i.e. change in behaviour between two groups. These two groups are typically called control and treatment groups. In this case, patients who paid their healthcare outstandings before Covid-19 appeared were in the control group, and patients who paid after Covid-19 were in the treatment group. The uplift behaviour of interest is the average collections from patients.